Freshly Printed - allow 10 days lead
Couldn't load pickup availability
Principles and Labs for Deep Learning
Provides complete methods and real-world labs for understanding and developing working Deep Learning tools for a variety of applications
Shih-Chia Huang (Author), Trung-Hieu Le (Author)
9780323901987
Paperback, published 25 June 2021
366 pages, 100 illustrations (50 in full color)
27.6 x 21.5 x 2.3 cm, 1 kg
Principles and Labs for Deep Learning provides the knowledge and techniques needed to help readers design and develop deep learning models. Deep Learning techniques are introduced through theory, comprehensively illustrated, explained through the TensorFlow source code examples, and analyzed through the visualization of results. The structured methods and labs provided by Dr. Huang and Dr. Le enable readers to become proficient in TensorFlow to build deep Convolutional Neural Networks (CNNs) through custom APIs, high-level Keras APIs, Keras Applications, and TensorFlow Hub. Each chapter has one corresponding Lab with step-by-step instruction to help the reader practice and accomplish a specific learning outcome. Deep Learning has been successfully applied in diverse fields such as computer vision, audio processing, robotics, natural language processing, bioinformatics and chemistry. Because of the huge scope of knowledge in Deep Learning, a lot of time is required to understand and deploy useful, working applications, hence the importance of this new resource. Both theory lessons and experiments are included in each chapter to introduce the techniques and provide source code examples to practice using them. All Labs for this book are placed on GitHub to facilitate the download. The book is written based on the assumption that the reader knows basic Python for programming and basic Machine Learning.
1. Introduction to TensorFlow2.02. Regression Problem3. Binary classification problem4. Multi-category Classification Problem5. Training Neural Network6. Advanced TensorFlow2.07. Advanced TensorBoard8. Convolutional Neural Network Architectures9. Transfer Learning10. Variational Auto-Encoder11. WGAN-GP12. Object Detection
Subject Areas: Biomedical engineering [MQW]
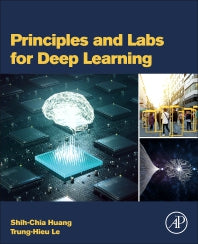